Enhancing Business Efficiency with Labeling Tool Machine Learning
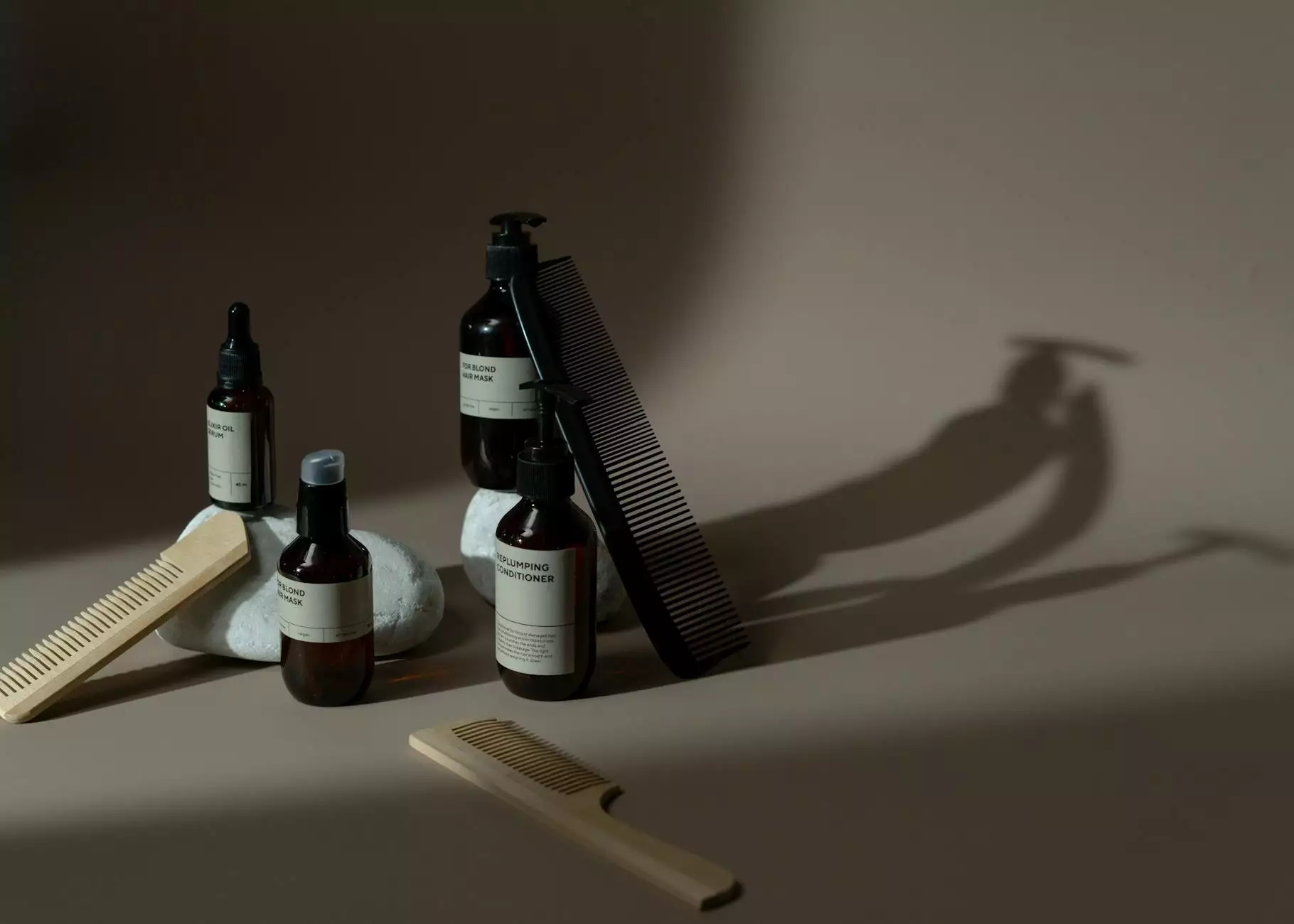
Understanding the Power of Machine Learning in Data Annotation
In today's rapidly evolving digital landscape, the integration of labeling tool machine learning is revolutionizing the way businesses handle data annotation. As organizations generate and collect vast amounts of data, proper structuring and labeling become crucial for deriving meaningful insights. Machine learning tools streamline this process, making it significantly more efficient and accurate.
What is Data Annotation and Why is it Important?
Data annotation provides the foundational groundwork for machine learning. It involves labeling data in a way that enables algorithms to understand and process information effectively. The applications are vast, ranging from computer vision tasks such as image recognition to natural language processing (NLP) applications that require text classification.
Without accurate annotation, machine learning models are likely to produce misleading or ineffective outcomes. Consequently, the role of advanced labeling tools has gained prominence.
The Role of Labeling Tools in Machine Learning
Labeling tools serve as the bridge between raw data and machine learning models, ensuring data is structured appropriately. These tools typically provide a user-friendly interface for annotators to tag data sets accurately, making them indispensable in the data annotation platform landscape.
Key Features of an Effective Labeling Tool
When selecting a labeling tool, businesses should look for several key features:
- Ease of Use: A user-friendly interface that minimizes the learning curve for annotators.
- Automation Capabilities: Features that leverage AI to suggest labels or automate repetitive tasks.
- Collaboration Tools: Capabilities that enable teams to work together seamlessly, regardless of location.
- Customizability: The ability to tailor the tool to specific project needs and requirements.
- Integration: Compatibility with existing data management and machine learning platforms.
Benefits of Using Machine Learning Labeling Tools
The benefits of incorporating labeling tool machine learning into business processes are manifold:
1. Increased Efficiency
Manual annotation is time-consuming and prone to error. Advanced labeling tools harness machine learning to automate large portions of the annotation process, significantly reducing the time needed for data preparation.
2. Enhanced Accuracy
By minimizing human intervention, machine learning tools reduce subjective biases, leading to more accurate and consistent data labeling outcomes. This accuracy is critical for training reliable machine learning models.
3. Scalability
As the volume of data grows, businesses can easily scale their annotation efforts by utilizing machine learning tools that can handle large datasets efficiently. This scalability ensures that organizations can keep pace with their data requirements.
4. Cost-Effective
Investing in an efficient labeling tool can lead to significant cost savings in the long run. By decreasing the time spent on data processing and increasing the accuracy of outputs, organizations enjoy lower operational costs.
Choosing the Right Data Annotation Tool for Your Business
Selecting the right tool from the myriad of options available can be a daunting task. When evaluating potential labeling tools, consider the following aspects:
1. Industry-Specific Requirements
Different industries have unique needs when it comes to data annotation. Ensure that the labeling tool supports the specific requirements of your sector, whether it be healthcare, finance, or e-commerce.
2. Integration with Existing Systems
Choosing a tool that integrates seamlessly with your current systems can save time and resources. Check compatibility with your data pipelines and machine learning frameworks.
3. Support and Training
Robust support and training services ensure smooth onboarding and usage of the labeling tool. Look for providers who offer comprehensive documentation, tutorials, and customer support.
4. Pricing Models
Different tools come with various pricing structures. Evaluate the cost in relation to the features provided and your budget. Some tools offer pay-as-you-go models, while others may require a subscription.
Implementing Machine Learning in Your Data Annotation Strategy
As businesses increasingly adopt machine learning technologies, integrating it into data annotation strategies becomes imperative. Here’s how to do it effectively:
1. Define Objectives
Clearly outline what you want to achieve with your machine learning model. This clarity will drive the annotation process.
2. Prepare Your Data
Collect and clean your data to ensure quality input into your model. This step is crucial for effective machine learning applications.
3. Choose the Right Annotation Tool
As discussed earlier, select a tool that best fits your business needs, considering factors like scalability, cost, and feature sets.
4. Train Your Team
Equip your team with the necessary skills to operate the chosen labeling tool. Training is vital for maximizing productivity and the accuracy of outputs.
5. Monitor and Optimize
Regularly evaluate the performance of your labeling tool and the quality of your annotation. Continuous improvement is key to successful machine learning outcomes.
Future Trends of Labeling Tools and Machine Learning
The future of labeling tools and their integration with machine learning holds exciting possibilities:
1. Increased Automation
Expect to see an uptick in tools that offer advanced automation capabilities, allowing for even less manual intervention in the labeling process.
2. Greater Accuracy through AI
Continuous advancements in artificial intelligence will lead to more sophisticated labeling algorithms that can adapt and improve over time.
3. Enhanced Collaboration Features
With more businesses adopting remote work models, collaborative features in labeling tools will gain importance, allowing teams to work together effectively regardless of location.
4. Specialized Tools for Niche Industries
As different sectors have distinct needs, we can anticipate an increase in the development of specialized labeling tools tailored for specific industries.
Conclusion: Embracing Change for Business Growth
In conclusion, the integration of labeling tool machine learning into data annotation processes signifies a transformative shift for businesses. By adopting these innovative tools, companies can enhance their data handling capabilities, drive operational efficiencies, and ultimately, achieve significant growth. As the digital landscape continues to expand, leveraging sophisticated labeling tools has become more crucial than ever—empowering businesses to stay competitive and thrive.